News2023.10.20
Research taking us one step closer to fully self-driving cars
When Jens Henriksson started at Semcon in 2015, he hardly knew what AI was. Now he is defending his PhD thesis examining how we can ensure that deep learning models make the right decisions in situations where safety is critical.
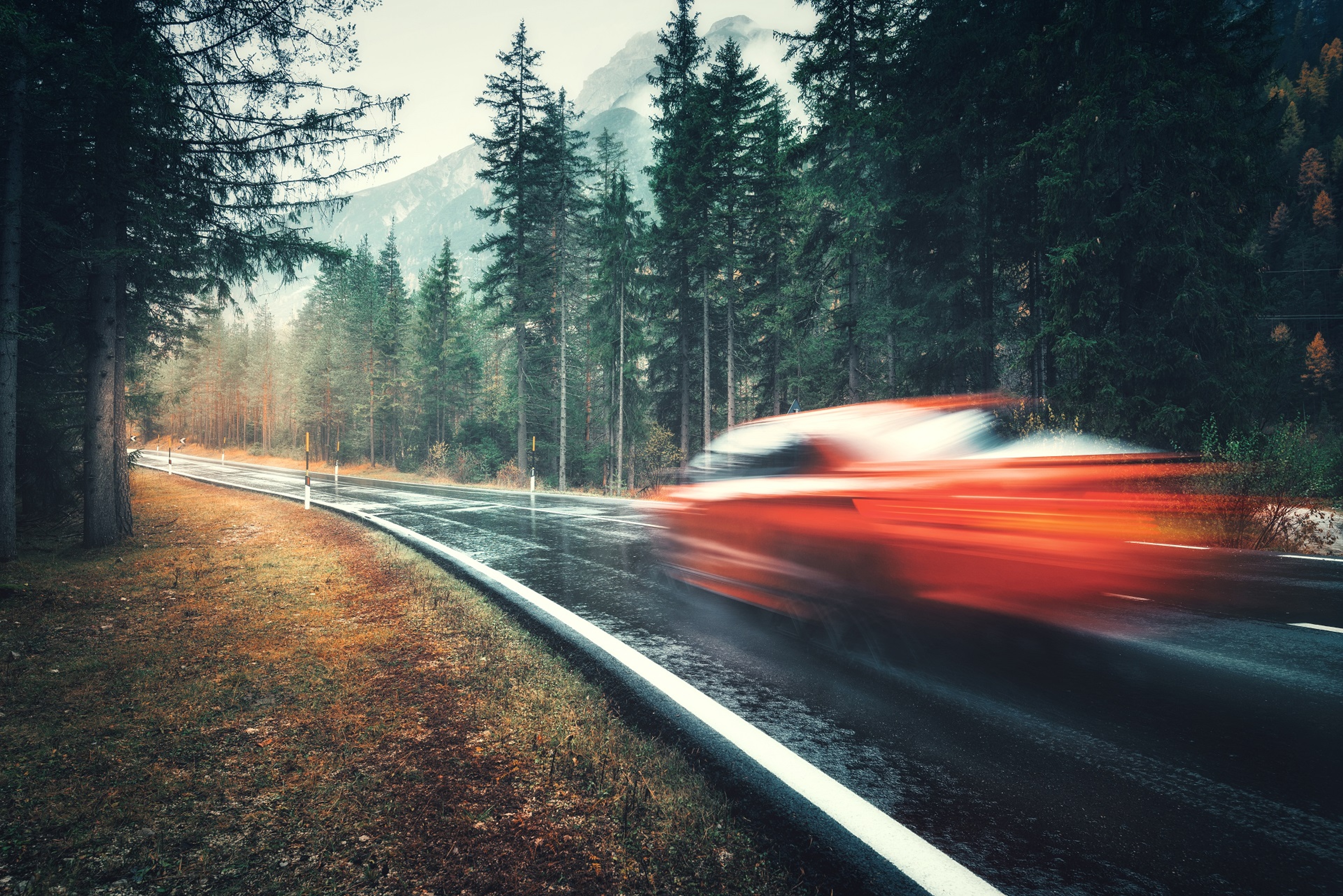
For a car to be driven entirely by computers, we need to be able to verify how secure the “self-driving” decision-making systems are. This is the primary challenge that the car industry is currently facing. Our current solution to this challenge, a human who keepstheir hands on the wheel when the car "drives itself". The irony of this “solution” is not lost on Jens who sums up the situation: “When it comes down to it, the [computing] models that handle the images from the car's cameras are so complex that you don't really know how they arrive at different decisions when they act on unfamiliar data.” Security in image management influences other important applications as well such as diagnostic medical imaging. “These limitations prevent these systems from being used and contributing more to improving the world. That's why this work is so interesting and important.” says Jens.
My thesis has studied how we can identify when a model based on deep learning works with unfamiliar data.
- Jens Henriksson, industrial PhD student at Semcon.
An important step in harnessing the full power of AI
Jens’swork has focused on image management, a process central to the core technology of self-driving cars and medicine. He has come up with a method to help security engineers assign security requirements to deep learning modelsand evaluate how they behave when working with unfamiliar data. Jens’s research is an important step towards using the full power of AI in several areas.
“Deep learning is used today in a number of familiar areas, for example in your smartphone to recognize your voice.The goal of mythesis has been to investigate how we can connect parameters from deep learning with verification and testing for safety-critical applications, such as self-driving cars.”
This also means that Jens had to investigate what additions are needed to verify deep neural networks.
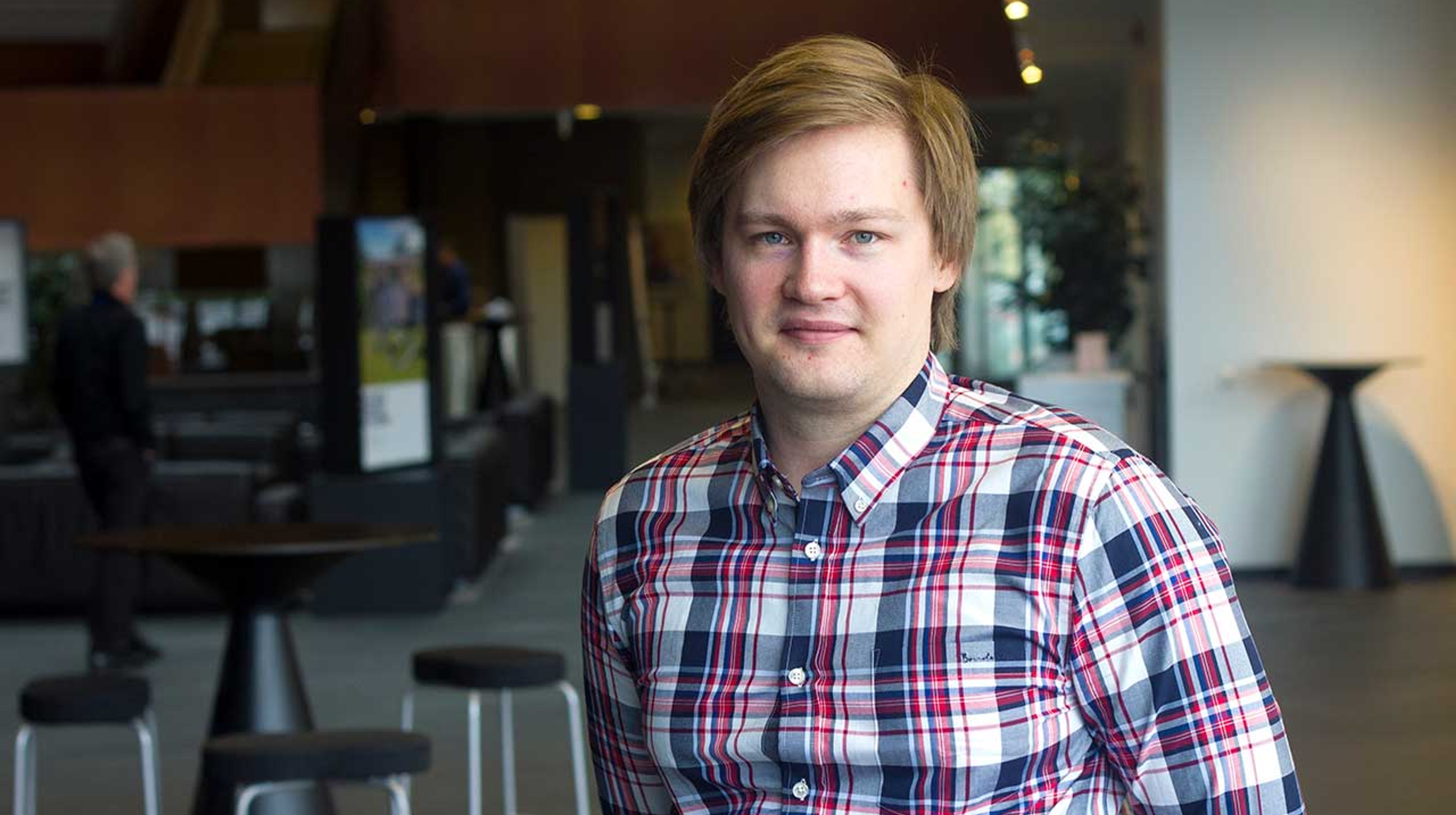
We need to know that the car is making safe decisions.
In a self-driving car, the model receives data from the car's cameras, the images are interpreted in real time and then form the basis of how the car behaves when you let it drive itself. Most of the time, everything runs smoothly, because the model is so accurate in correctly interpreting the images it is fed with. But what happens if it suddenly gets foggy? Or a light phenomenon occurs, which the model has not encountered before?
“Since current modelsare based on data from previous experiences, wecannot be sure that it makes the right decision in relation to safety when it encounters a completely new situation. This is because the image analysis model may not even be aware that there are risks [related to] the situation, which is difficult to verify due to the complexity of the models.” says Jens.
What Jens has come up with is a new analysis approach to combine deep learning and current analysis techniques used to manage new unknown images (outlier data). Creatingbuilding blocksfor use in deep learning areas where security is crucial.
“Our experiments show that the effect of [outlier] samples can be mitigated by extendingthe deep learning model with safety measures, i.e., measures that reduce the impact of undesiredbehavior. This thesis shows how to use a risk-coverage trade-off metric that connectsdeep learning performance with functional safetyrequirements.” The potential is huge, as the capabilities of AI models [for image analysis] far exceed standard traditional algorithms.
This is deep learning.
The human brain has a unique ability to handle and process information. Deep learning, or deep machine learning, is a method in AI inspired by how the human brain works. The technology is based on an AI model that can learn on its own to draw conclusions based on different types of data.
Read more about Jens’ research
On October 20, 2023, Jens Henriksson defended his thesis with the title