ArticleMobility
Federated learning for automotive
Creating sustainable data processing through decentralised learning and edge technology. Developing the next generation of vehicles with self-driving capabilities increases the need to handle massive amounts of data. A global automotive manufacturer selected Semcon to help define how to design its data processing to become more efficient and sustainable. And the answer is found in the latest technology.
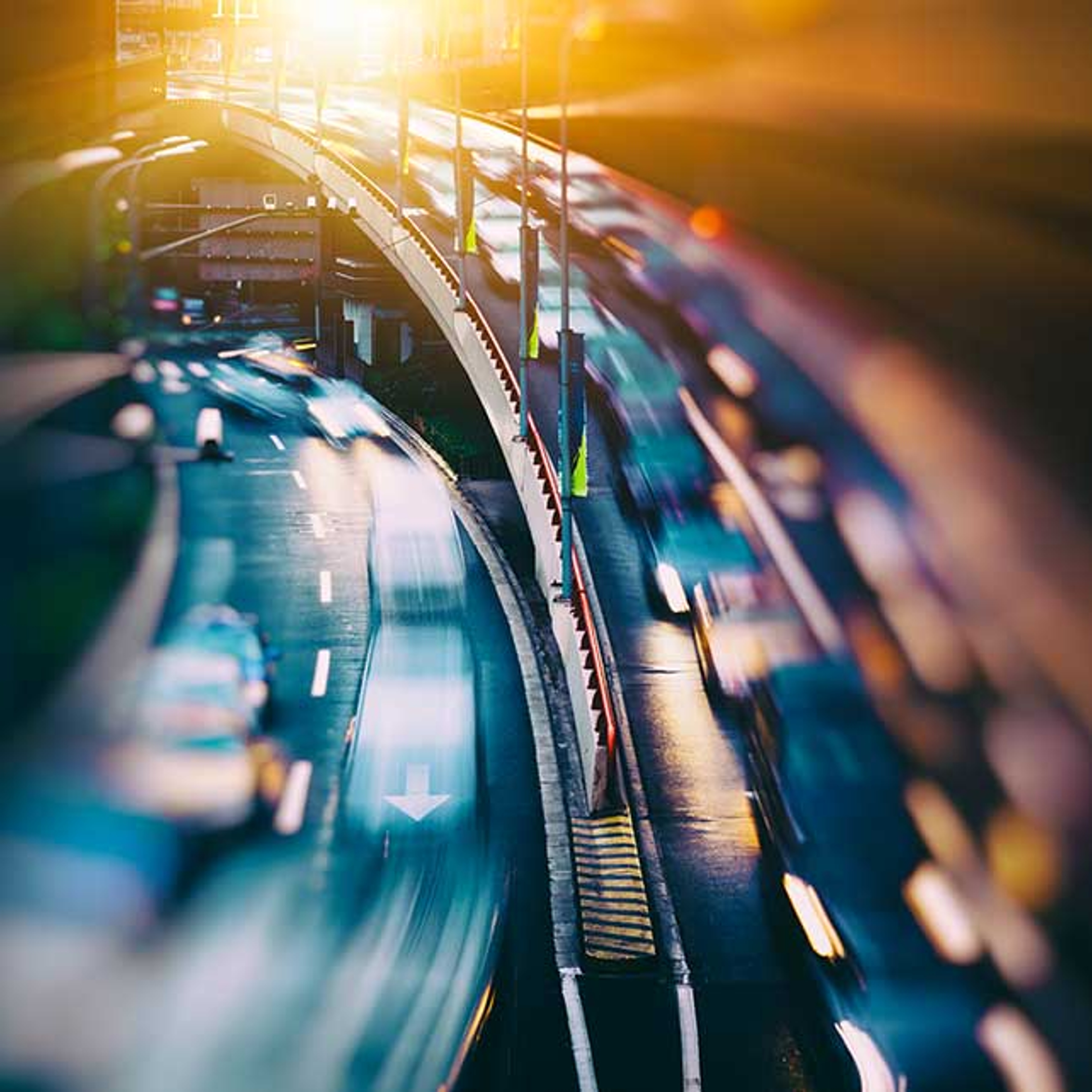
To develop safer and more intelligent vehicles with self-driving features, a massive amount of data needs to be gathered to train the system to achieve the desired functionality, such as in order to correctly perceive the traffic situation and the intentions of other road users for safe autonomous driving.
Today, most of the generated data is transferred from the vehicles to a central server for training the functionality. Since the amount of data is massive, this process adds extensive costs and is very time consuming.
Our main goal for this assignment is to define a sustainable data processing solution built on the latest technology for our customer.
– Mats Larsson, Team Manager Software & Emerging Tech at Semcon
Limited data transfer reduces both cost and energy use
By implementing new edge approaches such as federated learning, the vehicle data are only used locally to train the systems’ models, and only the results and learnings are transferred to the central server where they are aggregated. Evaluation is then carried out and improved functions can be transferred back to the vehicles by, for example, over-the-air updates.
”This approach will limit the data amount transferred to central learning and dramatically reduce the costs and energy use. These are important key factors in the further development of a sustainable infrastructure, which is needed for the next generation of vehicles”, says Mats Larsson and continues:
“Another great source of added value from distributing the data processing is that it simplifies privacy concerns (GDPR) as well as handling of data from restricted areas since data are instead handled locally. For example, location information and other private information can be used as input to the training in the vehicle but this information is not transferred back to the central system.”
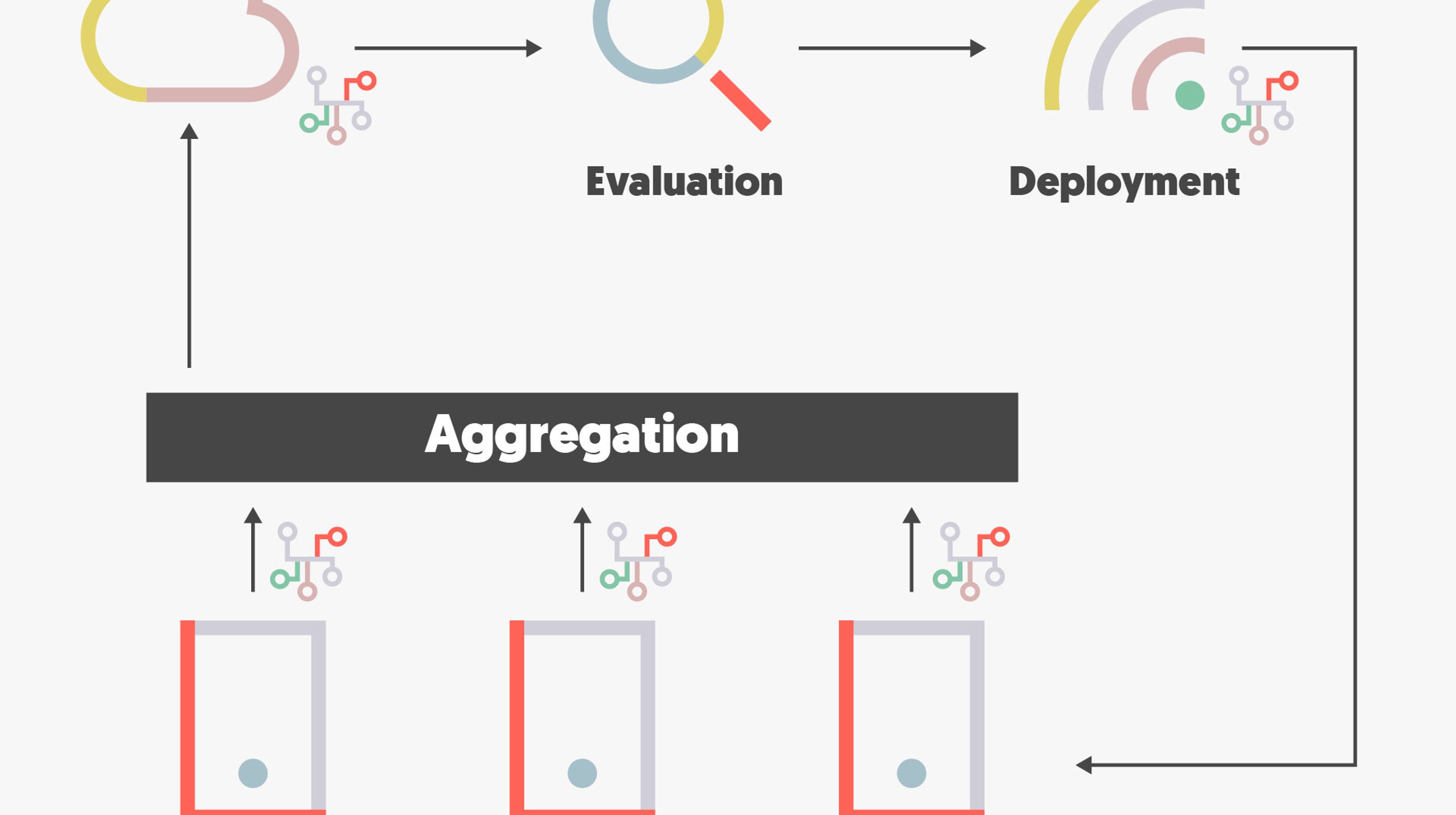
A state-of-the-art investigation in progress
In the initial stage of the project, Semcon’s experts are gathering top knowledge on how edge technology and decentralised learning can minimise data transfer. The work is performed in an agile approach with regular alignments and workshops together with the customer. As part of the investigation, interesting usage cases are defined and a number of them will be realized in , a test bed environment for proof of concept and comparisons.
Federated Learning in short
Federated learning is a machine learning approach that enables edge nodes to collaboratively learn a shared prediction function while keeping all the training data on the edge, which eliminates the need to store data centrally to carry out machine learning. Instead, the only information that is transferred consists of focused updates on the models that have been improved on the edge by the learning from its data.
Semcon’s expertise in this project consist of:
AI-driven data management
Previous experience in working with machine learning research projects (doctoral level)
Understanding of machine learning in distributed/federated contexts
Experience in developing software for embedded environments
Experience in working with cloud computing
Usage of decentralised learning techniques is applicable not only to the automotive sector but also to areas such as apps in mobile phones, digital health solutions, industry 4.0 smart manufacturing solutions, etc. And this is an approach that can help domains reduce data transmissions and build models on costly/private data while preserving data integrity.
If you are also searching for guides and solutions on AI-driven data management in general, read more on our website, or contact us to set up a meeting.